Natural language processing reveals information not captured by codes in electronic health records
13 November 2019
A group of researchers led by the UCL IHI’s Dr Anoop Shah explored whether free text in electronic health records may contain additional disease information beyond coded information.
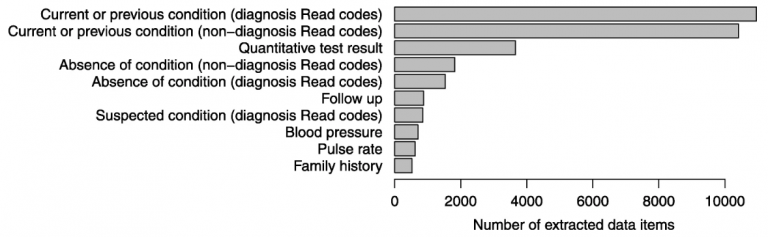
Electronic health records (EHR) contain a plethora of information about a patient such as their medical history, diagnoses and medications. EHRs allow access to evidence-based tools that can be used to make decisions about a patient’s care. They can also provide important data for clinical researchers. However, research studies typically use only the structured information (codes and numbers), but much of the information is in free text.
There is a lack of studies evaluating the extent to which free text provides additional information for research, particularly in primary care.
A group of researchers led by Dr Anoop Shah, at the UCL IHI, explored the contribution of free text in primary care to the recording of information about myocardial infarction (MI), including MI subtype, left ventricular function, laboratory results and symptoms; and recording of cause of death. This work has been published in the Journal of Biomedical Semantics.
The group used the CALIBER platform, which contains primary care data from the Clinical Practice Research Datalink (CPRD) linked to hospital admission data, the MINAP registry of acute coronary syndromes and the death registry. In CALIBER they randomly selected 2000 patients with myocardial infarction (MI) and 1800 deaths. They implemented a rule-based natural language engine, the Freetext Matching Algorithm, to analyse free text in the primary care record recorded within 90 days either before or after the MI, and on or after the date of death.
The group extracted 10,927 diagnoses, 3,658 test results, 3,313 statements of negation, and 850 suspected diagnoses from the MI patients. Inclusion of free text increased the recorded proportion of patients with chest pain in the week prior to MI from 19% to 27%, and differentiated between MI subtypes in a quarter more patients than structured data alone. Cause of death was incompletely recorded in primary care; in 36% the cause was in coded data and in 21% it was in free text. Only 47% of patients had exactly the same cause of death in primary care and the death registry, but this did not differ between coded and free text causes of death.
Among patients who suffer MI or die, unstructured free text in primary care records contains much information that is potentially useful for research and is not recorded in the structured data, such as symptoms, investigation results and specific diagnoses. Access to large scale unstructured data in EHRs (millions of patients) might yield important insights. Natural language processing to convert this information into a structured form can enrich primary care data at scale for research, and potentially yield population-based insights into early presentations of disease.
To read the paper visit the BMC website.
Shah, A.D., Bailey, E., Williams, T. et al. Natural language processing for disease phenotyping in UK primary care records for research: a pilot study in myocardial infarction and death. J Biomed Semant 10, 20 (2019) doi:10.1186/s13326-019-0214-4