Spotlight On: Dr Paul Expert, Lecturer in Health Informatics
24 February 2022
We speak to Dr Paul Expert, Lecturer in Health Informatics at UCL's Global Business School for Health, about his career, academic interests and why he's excited to have joined the UCL GBSH team.
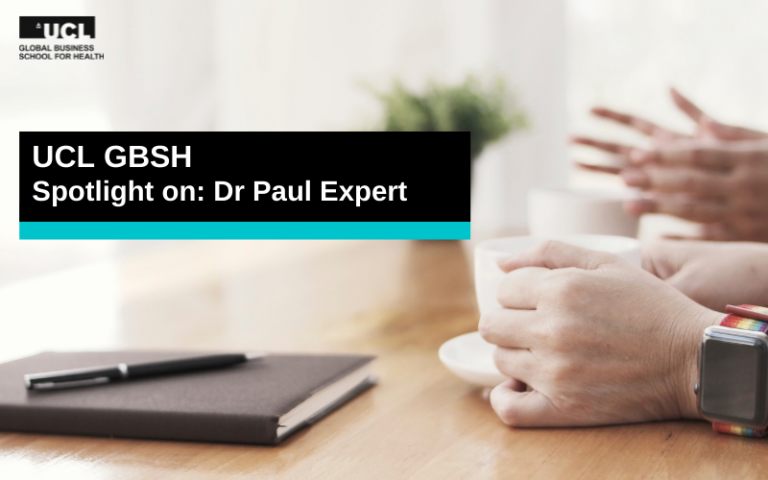
Tell me more about your background – It would be great to find out more about your education and career so far.
I had a formal upbringing in statistical physics in Geneva, but already during my undergraduate studies, I was interested in interdisciplinary endeavours. I solidified that approach during my PhD in the Complexity and Networks group at Imperial where I applied methods from statistical mechanics and network theory to neuroscience and sociology. From then on, I focused my research on the development of new methods that had applicability in real-life systems, with postdocs in the Centre for Neuroimaging Sciences at King’s College London, in the Department of Mathematics and in the Department of Primary Care and Public Health, both at Imperial. In each position, I developed and applied methodologies borrowing from network sciences, AI and statistical learning.
During my last post-doc in PCPH, I could see how I could combine methodological intellectual challenges that could provide actionable insights to improve healthcare delivery. Joining the UCL Global Business School for Health was a natural next step.
Why are you excited to be joining the UCL Global Business School for Health?
I enjoy interdisciplinary research environments. From a personal point of view, it helps me keep humble by showing me the extent of my ignorance, and from a research point of view, I believe cross-pollination is the only way to meaningfully tackle important societal problems that tend not to follow specialist boundaries but have rather a tendency to involve multiple fields of expertise.
Adding new dimensions and context to my research and proposing alternative approaches to other’s questions is my idea of collaborative research. I believe the range of expertise at GBSH, from methodology to law and health economy, will enable just that.
From a teaching point of view, it is extremely stimulating to be able to contribute to shaping future leaders in healthcare and provide them with the adequate tools to face the challenges of changing and improving healthcare delivery across the world.
What are you most excited to teach the first cohort of UCL GBSH students?
First of all, I’m particularly curious to meet the students and see who they are, what their expectations are, what they want to learn and where they want to go. I think it is particularly important for the students that will follow the health analytics route to be able to gain enough numeracy to grasp central ideas and mechanisms about health data analytics and AI methods in general. The ability not only to understand the “concept” of an approach but actually understand why it might or might not work in particular situations is of the utmost importance in a healthcare operational landscape that will rapidly become dominated by AI type analytics. Managers need to be able to understand what is under the hood to make correct decisions based on the analysis results they are provided with and avoid the garbage in, garbage out syndrome.
The second most important thing I want to teach the student is to think from a system-wide point of view. Interventions tend to be designed with a specific target, or type of patient in mind, without taking into consideration how changes might affect other treatments and patient pathways. Research is showing us that healthcare systems are complex systems and that we need to consider them as a whole rather than as a collection of independent silos. Access to large scale Electronic Health Records enables us to systematically take this approach.
Another aspect related to data-driven decision making, I want to convey to the students is that even if data analysis enables you to identify intervention that would yield positive changes in patient outcomes, they need to consider the human aspect of changes, both for healthcare workers and patients. Without them, there would be no data in the first place.
Is healthcare management an area more business schools should be exploring? If so, why?
The pandemic has shed light on shortcomings and limitations to current approaches to healthcare management at all levels, particularly the lack of preparedness against extreme events. The upside is that it accelerated the trend for healthcare providers to give access to their digital patient records for research. This is a boon for researchers, but also indirectly for healthcare managers who can see the benefit of data-driven approaches and numerate enough to understand and action them. Using digital records enables you to objectively, at least to the extent that the data gives a faithful representation of your healthcare system, audit the state of the healthcare system. At a hospital level, that might mean optimising patient pathways and identifying interventions to improve their outcomes. At a national level where data allows it, that might mean optimising rare diseases treatments.
I believe it’s not just healthcare management per se but rather data-driven management applied to healthcare that needs more widespread offering.
That is of course not to say that all the answers are in the data, data only helps you identify potential intervention is only the first step. Developing implementations by working closely with the healthcare professionals that will implement them and patients that will receive them is paramount for successful management.
What contribution do you want to bring to the school and future students?
I hope I will bring methodology with a smile. Often methods can be seen as dry, rigid mathematical formulas, and while there is some truth to that, they can also be brought to life and find new unexpected homes where they can flourish and help push the boundaries of our knowledge further.
To the students in particular, I want them to remember to never take anything at face value, particularly when there is a p-value or the tag “Machine Learning” next to it. One should always question where results come from and their interpretations, particularly in situations where decisions will have a real impact on people.