Integrating Data Science and Digital Humanities: what can possibly go wrong?
17 January 2018, 5:30 pm–6:30 pm
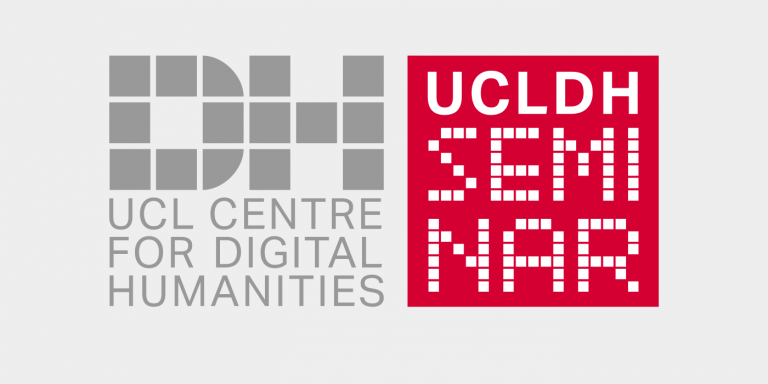
Event Information
Open to
- All
Organiser
-
UCL Centre for Digital Humanities
Location
-
UCL Centre for Digital HumanitiesGower StreetLONDONWC1E 6BTUnited Kingdom
The combination of qualitative and quantitative approaches (so-called "close reading" and "distant reading") is seen by many as the way to proceed in Digital Humanities (cf. DH Manifesto 2.0). The ambition to reach across the science/humanities divide is echoed in industry, with the emphasis on "thick data" (Wang 2013) to complement "big data", and in the Data Science community, with an increasing emphasis on human-centred Data Science, focussed on interpretability of machine learning models and a more active role of human input in algorithms (Chen et al. 2016).
In this talk Dr McGillivray will share her experience on conducting interdisciplinary research at the intersection between Data Science and Digital Humanities, and will stress the challenges, frustrations, and opportunities that lie ahead.
All welcome and there will be drinks and discussion after the talk. Attendance is free but we kindly ask that you register for the event.
Speaker
Dr. Barbara McGillivray is a research fellow at The Alan Turing Institute and the University of Cambridge. She runs the Data Science and Digital Humanities special interest group at The Alan Turing Institute. She holds a degree in Mathematics and one in Classics from the University of Firenze (Italy), and a PhD in Computational Linguistics from the University of Pisa (2010). Before joining the Turing Institute and the University of Cambridge, she worked as a language technologist in the Dictionary division of Oxford University Press and as a data scientist in the Open Research Group of Springer Nature.
Barbara McGillivray's research lies at the intersection between computational linguistics and historical linguistics and, more broadly, between Data Science and Digital Humanities. Her current research focusses on computational models of semantic change in historical texts. Her first book, Methods in Latin Computational Linguistics, was published by Brill in 2013 and her second book, Quantitative Historical Linguistics. A corpus framework, co-authored with Gard B Jenset, was published by Oxford University Press in 2017.
References
Nan-Chen Chen, Rafal Kocielnik, Margaret Drouhard, Vanessa Peña-Araya, Jina Suh, Keting Cen, Xiangyi Zheng and Cecilia R. Aragon. 2016. Challenges of Applying Machine Learning to Qualitative Coding. In CHI 2016 workshop on Human Centred Machine Learning (HCML 2016)
Several authors. Digital Humanities Manifesto 2.0. Available at http://humanitiesblast.com/manifesto/Manifesto_V2.pdf
Tricia Wang. 2013. Big Data needs Thick Data. Blog post available at http://ethnographymatters.net/blog/2013/05/13/big-data-needs-thick-data/ (last access 15/12/2017)