Computational Cancer Genomics Research Group
Group Leader: Dr Simone Zaccaria
Cancer Research UK Career Development Fellow
Group Members: CCG Members
Overview
Our CCG research group focuses on the design and development of computational methods to analyse tumour DNA sequencing data for understanding different cancer evolutionary processes. Particularly, one of our key goals is the understanding of the evolutionary histories and migration patterns of metastatic cancer cells. To achieve this goal, we focus on the analysis of the cutting-edge single-cell data that we generate using single-cell whole-genome sequencing technologies, which enables the whole-genome sequencing of thousands of individual cells in parallel from the same tumour. The novel features of these revolutionary data have the potential to reveal novel cancer evolutionary and metastatic insights at unprecedented resolution, but they make their analysis particularly challenging. To address the challenges of this new era of cancer genomics, we develop computational methods that are based on rigorous mathematical models and algorithms. Combining innovative biological technologies with formal consolidated methodologies is the key to enable us to address these important unanswered questions and identify the related opportunities for clinical translation.
Details of Research
Recent sequencing technologies provide an effective way to investigate the cancer evolutionary process, with critical impact on both diagnosis, prognosis, and treatment. Therefore, a large amount of multi-dimensional and multi-omics data are routinely produced by cutting-edge technologies, including single-cell, bulk, and spatial sequencing as well as liquid biopsies and sequencing of metastases. While these data offer an unprecedented view of tumor evolution, the combination of such technologies with formal consolidated methodologies is the key to realize their full potential.
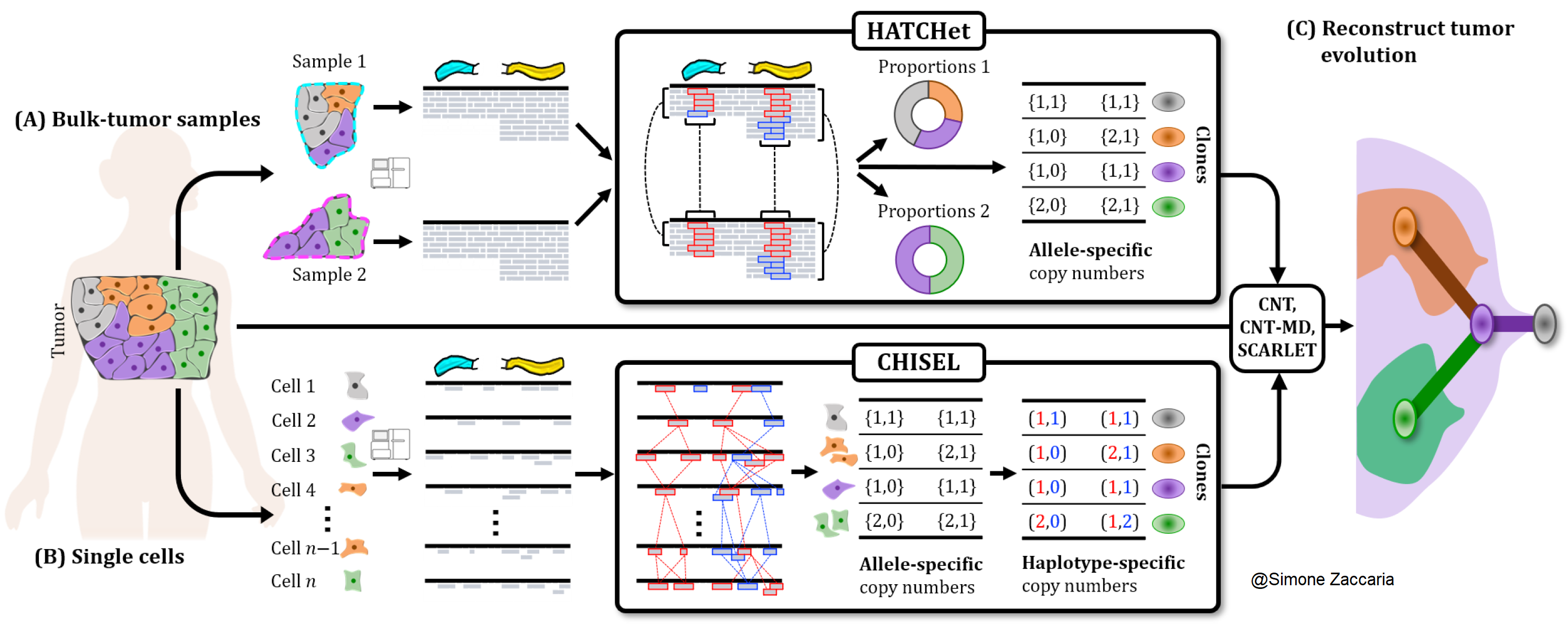
The goal of our lab is to design and develop computational methods that leverage the features of the most recent sequencing technologies to investigate the complex tumor evolution and heterogeneity. Such methods are thus based on rigorous mathematical models and algorithms that we specifically design for analyzing the data produced by different technologies. By framing biological questions as computational problems, we enable the integration of multiple sources of information into the solutions, revealing novel insights about the cancer evolutionary process.
- Open positions
We are seeking a collaborative and self-motivated computational cancer genomics post-doc to work on metastatic evolution and migrations using novel single-cell whole-genome DNA sequencing (scWGS) data. Please send your expression of interest, informal enquiries, and questions to Simone Zaccaria at s.zaccaria@ucl.ac.uk. Details below:
- Computational Cancer Genomics postdoc (Full Job description and details at: https://t.co/3RuniFk5kv)
- Description: The CRUK-funded project will focus on the design and development of computational methods and algorithms to analyse metastatic evolution and migrations using newly generated scWGS data. The unique combination of cutting-edge revolutionary scWGS technologies with the TRACERx/PEACE availability of longitudinal extensive metastatic tissue sampling (>40 samples per patient), clinical annotations, and orthogonal multiomics datasets makes this project an unprecedented opportunity to investigate complex metastatic processes and to demonstrate the importance of formal consolidated computational methodologies.
- Location: The successful candidate will be based within the Computational Cancer Genomics research group at the UCL Cancer Institute. The research will be conducted at both the UCL Cancer Institute and the Francis Crick Institute in close collaboration with the renowned and established multi-disciplinary team of computational biologists, cancer evolutionary biologists, and lung cancer translational researchers within the TRACERx and PEACE studies.
- Duration: The post is funded for 3 years in the first instance with a start date after 1st July 2022 and with the possibility of extension for another 2 years.
- Requirements: The successful applicant should have a related PhD (or equivalent experience) and a proven track record in cancer computational biology, have previous experience with NGS data analysis, be fluent in the Python programming language, and is expected to have strong skills in the field of genomics and desirably one or more of the following: cancer and evolutionary biology, statistics, algorithmics, and mathematics. Prior experience in the development of algorithms to identify genomic variants from DNA sequencing data and investigate their evolution is particularly desired.
- Application: Applications are now open and applicants should apply online through UCL portal (link: https://bit.ly/3zby6t6) by 26th June (currently a fixed deadline).
- Bioinformatics postdoc (position expected to open in Late 2022/Early 2023)
- Description: The CRUK-funded project will focus on the development of bioinformatics pipelines (for example using Nextflow) and statistical analyses to investigate metastatic evolution and migrations using newly generated scWGS data. The unique combination of cutting-edge revolutionary scWGS technologies with the TRACERx/PEACE availability of longitudinal extensive metastatic tissue sampling (>40 samples per patient), clinical annotations, and orthogonal multiomics datasets makes this project an unprecedented opportunity to investigate complex metastatic processes and to demonstrate the importance of formal consolidated computational methodologies.
- Location: The successful candidate will be based within the Computational Cancer Genomics research group at the UCL Cancer Institute. The research will be conducted at both the UCL Cancer Institute and the Francis Crick Institute in close collaboration with the renowned and established multi-disciplinary team of computational biologists, cancer evolutionary biologists, and lung cancer translational researchers within the TRACERx and PEACE studies.
- Details: TBA
- Application: Applications are expected to open between Late 2022 and Early 2023.
- Computational Cancer Genomics postdoc (Full Job description and details at: https://t.co/3RuniFk5kv)
- Selected Publications
The full list of publications is available in Google Scholar, ORCID, or Scopus as well as here in Our Publications. Author order generally follows convention in biology, with First Author listed first, and additionally: † indicates Joint First authorship, and * indicates corresponding authorsip.
- Zaccaria, S., Raphael, B.J. Characterizing allele- and haplotype-specific copy numbers in single cells with CHISEL. Nature Biotechnology (2020). https://doi.org/10.1038/s41587-020-0661-6
- Zaccaria, S., Raphael, B.J. Accurate quantification of copy-number aberrations and whole-genome duplications in multi-sample tumor sequencing data. Nature Communications 11, 4301 (2020). https://doi.org/10.1038/s41467-020-17967-y
- Satas, G.†, Zaccaria, S.†, El-Kebir, M.†, & Raphael, B. J. DeCiFering the elusive cancer cell fraction in tumor heterogeneity and evolution. Cell Systems, 12(10), 1004-1018 (2021). https://doi.org/10.1016/j.cels.2021.07.006
- Myers, M.A.†, Zaccaria, S.†, Raphael, B.J. Identifying tumor clones in sparse single-cell mutation data, Bioinformatics 36: i186–i193 (2020). Accepted and presented at ISMB 2020. https://doi.org/10.1093/bioinformatics/btaa449
- Satas, G., Zaccaria, S., Mon, G., & Raphael, B. J. SCARLET: Single-Cell Tumor Phylogeny Inference with Copy-Number Constrained Mutation Losses. Cell Systems, 10(4): 323-332 (2020). Accepted and presented at RECOMB 2020. https://doi.org/10.1016/j.cels.2020.04.001
- Zaccaria, S., El-Kebir, M., Klau, G. W., & Raphael, B. J. Phylogenetic copy-number factorization of multiple tumor samples. Journal of Computational Biology, 25(7): 689-708 (2018). Accepted and presented at RECOMB 2017. https://doi.org/10.1089/cmb.2017.0253
- Pirola, Y., Zaccaria, S.†, Dondi, R., Klau, G. W., Pisanti, N., & Bonizzoni, P. HapCol: accurate and memory-efficient haplotype assembly from long reads. Bioinformatics, 32(11), 1610-1617 (2016). Accepted and presented at ISMB-HITSeq 2015. https://doi.org/10.1093/bioinformatics/btv495
- Affiliations
- Collaborators
- Professor Charles Swanton, UCL Cancer Institute and the Francis Crick Institute
- Dr Nicholas McGranahan, UCL Cancer Institute
- Dr Mariam Jamal-Hanjani, UCL Cancer Institute
- Dr Nnenna Kanu, UCL Cancer Institute
- Dr Kevin Litchfield, UCL Cancer Institute
- Professor Ben Raphael, Princeton University
- Our Funders