New PhD studentship in energy and artificial intelligence with ERBE CDT
28 February 2020
The new 4-year studentship is part of the EPSRC-SFI Centre for Doctoral Training in Energy Resilience and the Built Environment (ERBE CDT), is sponsored by Igloo Energy, and starts September 2020.
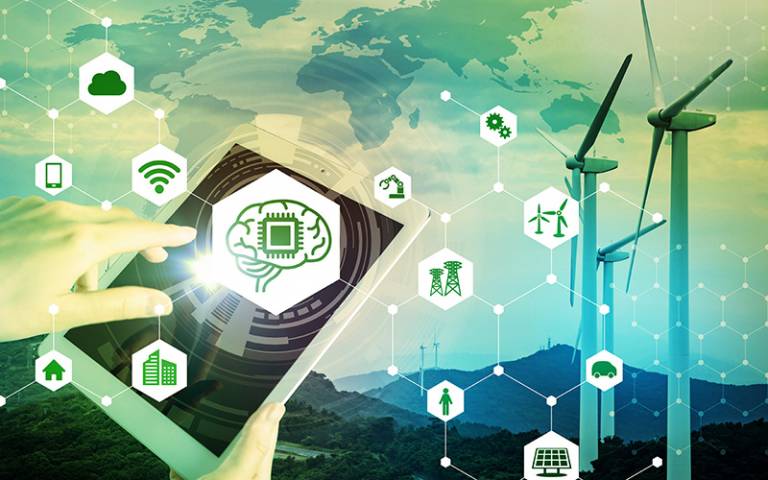
Localised high resolution forecasting for energy demand based on smart meter data
Sponsored by Igloo Energy
Supervisor
Dr Aidan O’Sullivan, UCL Energy Institute
Stipend
The studentship will cover UK course fees and an enhanced tax-free stipend of approx. £18,000 per year for 4 years along with a substantial budget for research, travel, and centre activities.
Dates
4 years starting September 2020
Industry Sponsor
Founded in 2017, Igloo Energy is an energy supplier with the mission to make their customers’ homes smarter, more efficient and cost less to run while helping the world get a cleaner energy system. Igloo provides customers with a mix of technology and by analysing data on how they use energy in their home, Igloo aim to reduce energy usage for the customer.
About the studentship
PhD in Energy and Artificial Intelligence
Using smart meter data provided by the partner utility company, this PhD will investigate the applicability of bottom-up forecasting models for energy demand trained on historical data from individual smart meters.
The availability of smart meter energy data is a significant asset for utilities businesses that offers the potential of significantly improving business operations while overcoming the challenges caused by the electrification of heat and transport and the growth of distributed generation.
One core business operation that could be improved with better customer information is the forecasting of demand (and distributed supply) at high spatio-temporal resolution. Demand forecasts are central to a utilities business and influence decisions on the purchasing of electricity and hedging of future prices which are a cost to the business. Failure to hedge future prices correctly and exposure to highly volatile balancing market costs have been a contributory factor in the failure of a number of utilities.
Studentship aims
Using smart meter data provided by the partner utility, this PhD will investigate how bottom-up forecasting models can be used to predict energy demand at a number of scales. These models will be trained on historical data from individual smart meters, and the forecasts will be aggregated at a local/regional level and compared with the accuracy of top down forecasts to assess what benefits exist and what level they occur.
Particular avenues of exploration could be:
- Spatial information allowing exploration of scenarios such as the impact of Locational Marginal Pricing (nodal pricing), a policy implemented in the United States and much discussed in the UK, on a utility based on its current portfolio of customers and the impact of the spatial distribution of its customers on profitability.
- The potential for balancing portfolios of customers, locally or within the suppliers base, and identifying locations of possible network constraints and substations likely to reach capacity with the uptake of electric vehicles.
- Design of an optimal trading agent for the hedging and purchasing of electricity given the portfolio of smart meter information is a likely final application of the research that would bring together a number of the individual strands of the project.
Methods
As a starting point, state-of-the-art ensembling methods will be tested as a benchmark before exploration of the potential for deep learning methods in particular Long-Short-Term-Memory Neural Networks to improve results. Reinforcement learning and agent-based models will also be explored throughout the course of the project.
Person specification
The research will require candidates with a background in a quantitative field such as maths, physics, computer science or engineering and an interest in the energy sector and decarbonisation. Experience in one or more of the following would be an advantage: statistics, machine learning, AI and programming.
Candidates should have a Master’s degree and / or a first or upper-second class Bachelor’s degree.
Applicants must also meet the minimum language requirements of UCL.
Application procedure
How to apply
Please submit a pre-application by email to the UCL ERBE Centre Manager (bseer.erbecdt@ucl.ac.uk) with Subject Reference: 4year PhD in Localized high resolution forecasting
The application should include the following:
- A covering letter clearly stating which project you wish to apply for, your motivation, and your understanding of eligibility according to the EPSRC guidelines
- CV
- Names and addresses of two academic referees
- A copy of your degree certificate(s) and transcript(s) of degree(s)
Applicants should meet the EPSRC eligibility criteria.
Deadline for applications: Thursday, 30 April 2020 23:59 (UK time)
Interview process
The interview panel will consists of the project’s academic supervisor at UCL, a representative of the industrial sponsor and a representative of the ERBE CDT Academic management.
Only shortlisted applicants will be invited for an interview.
Following the interview, the successful candidate will be invited to make a formal application to the UCL Research Degree programme. For further details about the admission process, please contact: bseer.erbecdt@ucl.ac.uk.
For any further details regarding the project, contact Dr Aidan O’Sullivan on aidan.osullivan@ucl.ac.uk.
You will be undertaking this project in UCL at the main (Bloomsbury) campus as part of the new EPSRC-SFI Centre for Doctoral Training in Energy Resilience and the Built Environment (ERBE CDT). This is a collaboration between UCL, Loughborough University and Marine and Renewable Energy Ireland (MaREI). For more information please visit the ERBE CDT website.