CASA Working Paper 215
Unpacking the Nuances of London’s Neighbourhood Change & Gentrification Trajectories
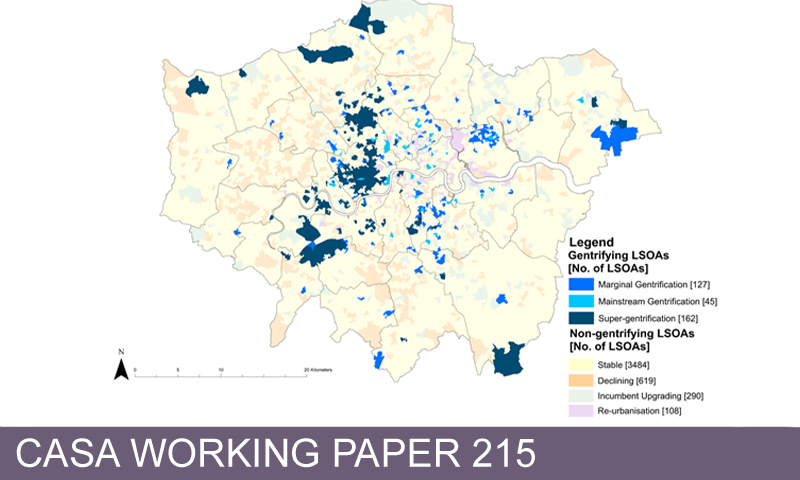
13 January 2020
Amid the simmering global economic uncertainties and geopolitical instabilities of our present day, deepening social inequalities have remained a constant in our contemporary urban landscapes. Particularly within global cities like London, the disparities have become
increasingly apparent. The gravity of the situation is underscored by recently published reports suggesting London’s position as home to not only the majority of the UK’s richest 1%, or indeed the 0.1%, but also a large proportion of its urban poor. In spite of such polarisations, a sense of indifference towards the needs of the average Londoner seemingly prevails throughout London’s hyper-commodified housing market, which is typified by sky-rocketing property prices and a chronic undersupply of truly affordable housing options. Gentrification, emanating from the nexus of such trends, has been endemic in London; propelling socio-spatial transformations in localised neighbourhoods and displacing incumbent residents. Despite gentrification manifesting in more accentuated and diversified ways than ever before, recent research has only been tepid in honing the critical edge necessary for effectively distinguishing gentrification from other forms of neighbourhood change and to rigorously dis-aggregate gentrification’s nuances. This has consequently limited the depth of contributions that academia can make towards robust policy-making.
Addressing such issues, this paper employs a novel empirical approach, that synergises recent advancements in Machine Learning, new data sets and spatial analysis techniques, to systematically examine the variegated past and future trajectories of neighbourhood change
across London. The nature of gentrification’s mutations and its spatial patterning in London are further extracted using a combination of Principal Component Analysis, K-Means clustering and in-depth spatial analysis. Machine Learning is subsequently adopted to model gentrification’s observed trends and predict its future frontiers; thereby offering policy-makers unprecedented and highly-contextualised insights into gentrification’s projected dynamics and geographies.
Authors: Joshua Yee & Adam Dennett
Download CASA Working Paper 215 (file size 11.5MB, file format PDF)