Student profiles
23/24 academic year
Ming Liang Ang
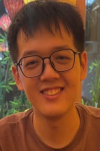
Ming Liang is currently pursuing his PhD in the algorithmic and theoretical foundations of machine learning. He is interested in understanding the conditions under which transfer learning effectively occurs and hopes to elucidate key design principles that can enable more effective transfer learning to take place, especially in foundation models. Prior to the Foundational AI CDT, he obtained a degree in mathematics from the National University of Singapore (NUS) and was awarded the Ho Family Prize for being the top applied mathematics student of his cohort.
Sierra Bonilla
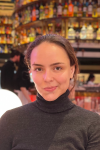
Neural Rendering
Sierra Bonilla is interested in neural rendering frameworks for surgical scene reconstruction. Prior to this, Sierra completed a Master's in Medical Physics & Biomedical Engineering at UCL and holds a Bachelor's in Bioengineering and Mathematics minor from the University of Washington. With four years of prior experience in academic and industrial healthcare research, Sierra is dedicated to pushing the boundaries of AI for healthcare problems and beyond. https://sierrabonilla.com/
Natalia Burton

I'm particularly interested in neuro-inspired AI and AI safety. My research focus in the development of bio-plausible and energy efficient architectures capable of generalising and succeeding in goal-oriented tasks whilst minimising power consumption. I have a degree in Electronic Engineering and a Master's in Business Management and Engineering. Prior to joining the Foundational AI CDT, I held a number of positions in tech-driven companies, most recently as Solutions Architect helping financial institutions to leverage the power of graph analytics and contextual data in uncovering fraud and other financial risks.
Milo Carroll
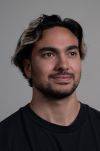
I am currently researching generative models for human motion synthesis. My primary objective is to understand how motion data can serve as a useful foundation for controlling physically simulated characters and robots in a reinforcement learning context. Additionally, I am exploring ways to efficiently repurpose these generative policies for downstream tasks, such as incorporating vision and language. My research primarily focuses on the fields of Reinforcement Learning, Robotics, and Deep Generative Models.
Francesca Channon

Francesca Channon is currently pursuing her PhD, focusing on the application of machine learning techniques to enhance energy efficiency in the built environment. Her research aims to address the limitations of traditional energy assessment methods by integrating supervised learning, probabilistic approaches, and physics-informed machine learning. Prior to her PhD, Francesca obtained a degree in Mathematics from Warwick University, and an MSc in Statistics, and MSc in Applied Biotechnology and Biosciences from Imperial College London. She has industry experience in sustainable investment due diligence and alternative investments at J.P. Morgan Private Bank, and consulted on ESG-related topics at Tellus Matrix. Francesca's research is supervised by Professor Tomaso Aste and Professor John Shawe-Taylor.
George Drayson

Natural Language Processing and Generative AI
George is a PhD student at the Foundational AI CDT at University College London, supervised by Professor Lampos. George is interested in Natural Language Processing and Generative AI and their applications in healthcare. Prior to starting his PhD, he obtained a MEng in Engineering Science from the University of Oxford. See georgedrayson.com for more details.
Rares Dolga
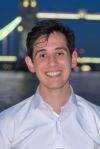
Rares is interested in probabilistic machine learning largely focusing on methods for efficient language generation. Prior to his PhD, he was a quantitative developer and then an AI researcher at JP. Morgan where he worked on NLP problems in finance. Rares obtained an MEng in computer science from UCL in 2020.
Ahmet Guzel

Ahmet Guzel is interested in confluence of AI, computational displays, and computer graphics. His primary pursuit involves the crafting of advanced deep learning techniques to amplify realism and interactivity within graphical displays. A significant part of his research revolves around the utilization of differentiable ray tracing for optical systems, aiming to seamlessly merge it with artificial intelligence, thereby enhancing both computational optics and the overall visual realism of computer-generated graphics through neural rendering. Under the mentorship of Dr. Kaan Aksit at the Computational Light Laboratory, Ahmet delves deeper into these intersecting domains, continually expanding his expertise. He holds an MSc in Artificial Intelligence from Leeds University and possesses a rich background in computational engineering, notably in the motorsports arena. https://aguzel.github.io/
Nathan Herr
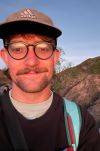
Machine Learning, Deep Learning, Natural Language Processing, Reinforcement Learning, Robotics, Multi-agent (Swarm), Cooperative AI, Ethical AI, AI for Health, Security, Education, Finance, Sport, Wildlife
I am passionate about using AI to benefit humanity. Particularly, enabling natural cooperation and coordination between AI agents and humans in all types of tasks. Outside of academia, I love staying active and being outdoors, soaking up all the sun the UK has to offer.
Hongyu Lin

Hongyu Lin is a PhD student at the CDT in Foundational AI, supervised by Professor Tomaso Aste and Professor John Shawe-Taylor. His research interests lie in statistical learning theory and causality. Prior to the PhD, he obtained a BSc in Mathematics and Physics from UCL, an MAST in Applied Mathematics from the University of Cambridge, and worked as a data scientist in the renewable energy industry.
Varsha Ramineni
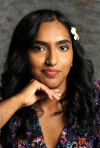
AI Bias and Fairness, Synthetic Data
Varsha is a PhD student and DeepMind scholar in Artificial Intelligence (AI) at UCL, supervised by Prof. Emine Yilmaz and Prof. David Barber. She is passionate about bridging the technical and societal aspects of AI systems through interdisciplinary research, with particular interest in AI bias and fairness, and synthetic data. Varsha holds an MSc in Statistical Science from Oxford University and a BSc in Mathematics from Warwick University. Before joining the PhD program, she worked in mental health research, where she applied Bayesian adaptive design techniques to evaluate a digital intervention for healthcare staff experiencing intrusive memories of traumatic events.
Leonie Richter

I am interested in reward models, deception and reinforcement learning from human feedback, specifically in the context of LLMs. I would like to contribute to the problem of aligning powerful artificial models with human values.
Prior to my PhD, I completed an internship at the Center for Human-Compatible Artificial Intelligence in Berkeley and worked as a Machine Learning Engineer. Before that, I completed my MSc in Artificial Intelligence at Imperial College London.
Romy Williamson

Geometry Processing
Romy is interested in the use of neural networks to represent smooth geometry, as an alternative to discretisations such triangle meshes. Prior to the PhD she earnt her BA in Mathematics from the University of Oxford and her MSc in Computer Graphics, Vision and Imaging from UCL. Her current research focuses on smooth spherical parametrisations and the optimisation of distortion-minimising homeomorphisms between closed surfaces - for example, for the purpose of texture transfer. She works in the UCL Smart Geometry Processing Group under the supervision or Prof. Niloy Mitra.
22/23 academic year
William Bankes

I am broadly interested in studying probabilistic machine learning and how uncertainty quantification can enable machine learning models to make more intelligent decisions.
Prior to my PhD I spent two years working as a Machine Learning Engineer in Canary Wharf before completing an MSc in Computational Statistics and Machine Learning at UCL.
David Chanin

David studies commonsense reasoning and argumentation, and is interested in exploring ways to merge symbolic reasoning and knowledge representation with deep learning. Prior to starting his PhD, David worked as a software engineer, and holds degrees from Stanford University and Georgia Tech.
Zonghao (Hudson) Chen
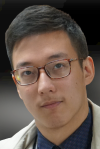
Hudson (Zonghao) is a Ph.D. candidate at the Center of Foundational Artificial Intelligence of University College London, supervised by François-Xavier Briol and Arthur Gretton. Prior to his PhD, he obtained the bachelor's degree from the department of Electronic Engineering, Tsinghua University. His research interests center around probabilistic modeling and causal inference. For more details, please check out https://hudsonchen.github.io/.
Gbetondji Dovonon

Gbetondji is broadly interested in deep learning, causality and graph theory, with the goal of designing more reliable and interpretable supervised learning systems that explicitly build on domain knowledge. He is a student at UCL and part of the ELLIS PhD program under the supervision of Matt Kusner and Michael Bronstein.
Jianwei Liu
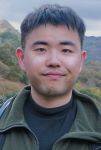
Reinforcement Learning, Legged robots, Lifelong learning, Neuromorphic learning
Jianwei is interested in leveraging ideas from neuromorphic learning to produce more efficient and robust legged robot systems, that can adapt to different environments/conditions. Prior to starting his PhD, Jianwei obtained a MEng in Biomedical Engineering from Imperial College London, a MSc in Robotics and Computation from UCL, and worked in industry as a robotics software engineer on off-shore NDT inspection robots and construction robots.
Hossein A. (Saeed) Rahmani

Conversational AI, Responsible IR, Recommender Systems
Hossein (aka Saeed) is a Ph.D. student in Foundational Artificial Intelligence at UCL AI Centre working on Fairness and Bias in Conversational Systems. Prior to starting his Ph.D., he received his MSc from the University of Zanjan, where he was working on context-aware recommender systems. His research interests lie in Information Retrieval, Recommender Systems, and Responsible AI. See https://rahmanidashti.github.io/ for more details.
Stuart Shanks
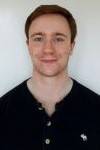
Reinforcement Learning, Continual Learning, Legged Robots
Stuart is interested in the intersection of optimal control and machine learning for robotics. He is supervised by Dr Dimitrios Kanoulas and Dr Carlo Ciliberto, and is working in the Robot Perception and Learning (RPL) Lab. He is particularly interested in continual learning algorithms for use on legged robots.
Hengyi Wang

Hengyi Wang is a PhD student at CDT in Foundational AI at UCL, primarily supervised by Prof. Lourdes Agapito. His research topic is "Neural Surface Reconstruction from Video". He is particularly interested in designing learning-based algorithms for real-time surface reconstructions from visual signals. Prior to starting his PhD, he obtained a BSc in Telecommunications Engineering with Management from the Queen Mary University of London and an MSc in Computer Graphics, Vision, and Imaging from University College London. For more information please check https://hengyiwang.github.io/
Jiayi Wang
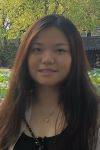
Jiayi Wang is a first-year PhD student with broad interests in artificial intelligence. She is currently working on natural language processing and adversarial machine learning.
Lorenz Wolf
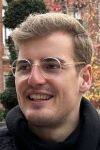
Multi Agent RL, Generative Modelling, Unsupervised Learning
Lorenz is generally interested in Multi Agent Reinforcement Learning, Generative Modelling, and Unsupervised Learning, and he is supervised by Mirco Musolesi. Prior to his PhD, Lorenz obtained his MSc in Statistics and BSc in Mathematics from Imperial College London.
21/22 academic year
Rokas Bendikas

Autonomous surgical robotics
I am a PhD student at University College London, working in the field of Surgical Robot Control and Robot Learning. My research project is focused on ‘Learning priors of high-level and low-level motion representations for autonomous robot control’. Therefore, I am interested in answering questions: ‘what perception modalities provide the optimum medium for an agent to learn’ and ‘how to learn complex behaviours that allow tackling long-horizon, multi-staged objectives in surgical setting’. During my Master’s studies, I completed my thesis at Dyson Robotics Lab, supervised by Prof. Andrew Davison. My project introduced imagination-augmented DQN, which allowed to learn optimal behavioural policy in dynamically complex environments. Previously I was a research assistant in Cardio-Electro Magnetic Research Group (CEMRG), investigating atrial fibrillation mechanisms through the means of Deep Learning and Computational Modelling. My work was supervised by Dr. Caroline Roney and Prof. Steven Niederer.
Jake (Harry) Cunningham

Research Area: Gaussian Processes and Climate Science
Jake is interested in understanding and improving uncertainty quantification in machine learning methods, focusing largely on Gaussian processes and kernel methods. He also has strong interests in applying statistical learning methods to problems in climate science. Prior to his PhD, he obtained an MEng in Engineering from the University of Oxford and MSc in Computing (Machine learning and AI) from Imperial College London.
Emilio Mcallister Fognini

Applications of AI to PDE's
Emilio is interested in using AI and machine learning to aid in the solving the equations that govern physical systems. His primary area of focus is efficiently and quickly solving general Helmholtz problems, with research interests in Inverse problems and applications of AI to scientific computing and physical systems. He obtained a Master of Mathematics from UCL.
Akbir Khan

Cooperative AI
Akbir is interested in studying techniques to ensure cooperative AI. He considers these problems primarily in the setting of multi-agent deep reinforcement learning and social dilemmas. He is advised by Tim Rocktäschel and Edward Grefenstette and affiliated with UCL DARK lab. For more information check https://www.akbir.dev
Aengus Lynch

Causal Inference
Aengus is interested in improving neural networks’ ability to generalise to unseen domains. He is advised by Ricardo Silva and associated with the Causal Machine Learning Group at UCL
Yao Lu

Natural Language Processing
Yao is interested in everything, especially human language model interaction (HLMI). The ultimate goal of his research is to create a unified communication interface between human and machine through human language.
Zak Mo
rgan
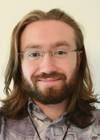
Computer Vision
Zak is a PhD student on the foundational artificial intelligence CDT at UCL, prevously having obtained his undergraduate masters in computer science at UCL. He is working under the supervision of Youngjun Cho and Sriram Subramanian in the UCL Interaction Center (UCLIC) primarily on the fields of computer vision and human-computer interaction.
Mariia (Masha) Naslidnyk

Gaussian processes and kernel methods
I am a first-year PhD student at the Foundational AI CDT at University College London, advised by Dr. François-Xavier Briol and Dr. Carlo Ciliberto. Prior to starting my PhD, I was a Machine Learning Scientist at Amazon Research in Cambridge, where I worked on Alexa question answering (2015-2019), and then on Gaussian processes for supply chain emulation (2019-2021). I graduated from Part III in Pure Mathematics at the University of Cambridge in 2014.
Daniel Ramos Macedo Antunes de Souza

Gaussian processes and fault detection
Daniel is broadly interested in the intersection of Bayesian machine learning models and the needs of their practitioners in the real world, more specifically, how theoretical benefits of Bayesian modelling translate or not into practical benefits. Before joining UCL, he obtained his MSc and BSc degrees in Computer Science at the Federal University of Ceará in Brazil.
Laura Ruis

Machine Learning
Laura is interested in understanding the generalization conditions of neural networks. See http://lauraruis.com for more details.
20/21 academic year
Fernando Acero
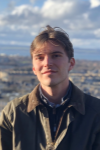
Reinforcement Learning, World Models, Robotics
I am interested in methods to enable reinforcement learning to be used to solve control problems in the real world in a robust, uncertainty-aware, and sample-efficient way, leveraging generative models of the world and model-based algorithms to enhance the capabilities of autonomous robots and beyond. Prior to starting my PhD I worked in control, perception, and autonomy at a drone startup, and obtained a MEng in Aeronautics with Spacecraft Engineering from Imperial College London.
Reuben Adams

Statistical Learning Theory, in particular PAC-Bayes
I completed an undergraduate degree in mathematics at Oxford in 2014 after which I was a maths teacher at Radley College for four years. Realising I wanted to return to academia and fancying a change, I moved to the Netherlands where I did a master's in applied mathematics at TU Delft, specialising in statistics. For my master's thesis, I worked with Peter Grünwald at CWI to develop new statistical tests that allow the user to decide when to stop collecting data based on the results already obtained, without losing statistical significance. It was through working with Peter that I learned about PAC-Bayes - a method to obtain rigorous generalization bounds for deep neural networks. This is the topic I am currently working on with my PhD supervisors Benjamin Guedj and John Shawe-Taylor. The goal of my PhD is to better understand the types of data-generating distributions that work well with PAC-Bayes, and whether we are lucky in that the distributions we generally come across in machine learning are of this type.
Yue Feng
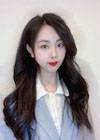
Conversational AI
Yue aims to design systems that robustly and efficiently learn to understand human languages and web data to the end of advancing artificial intelligence web service and web information processing. Her research interests lie in natural language processing, information retrieval, machine learning, and data mining.
Dennis Hadjivelichkov
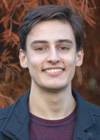
Self-Supervision for Robotics
Denis is working on bridging the gap between robotics and intelligence through machine learning. He is part of the Robot Perception and Learning Lab where he is currently dealing with self-supervised learning of object descriptors and affordance using cues provided by stereo vision, egomotion and kinematics.
Alex Hawkins-Hooker
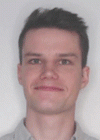
Protein design, deep generative models
Alex is broadly interested in the development of machine learning methods for the study of biological molecules. His PhD research focusses on the use of deep generative models for protein design and is supervised by David Jones and Brooks Paige.
J
ean Kaddour
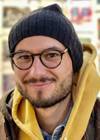
Causal Inference and Deep Learning
Jean works with Ricardo Silva and Matt Kusner on Causal Inference and Deep Learning. Jean obtained a MSc. in Advanced Computing at Imperial College London.
Causal Inference, Machine Learning
Oscar Key
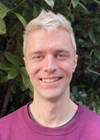
Machine Learning
Oscar is interested generally in probabilistic modelling and inference, including topics such as uncertainty estimation, robustness, and testing goodness-of-fit. He is motivated by applications to other areas of science, for example systems biology and climate modelling. Oscar is supervised by François-Xavier Briol and Marc Deisenroth, and his background is in computer science and software engineering.
Robert Kirk

Reinforcement Learning, Generalisation, Out-of-distribution Robustness, AI Safety
Robert is interested in reinforcement learning, especially generalisation and out-of-distribution robustness in reinforcement learning. He wants to understand how we can build reinforcement learning systems that generalise both capably and safely to unseen environments so that we can deploy them in the real world. He's supervised by Tim Rocktäschel and Edward Grefenstette as part of the UCL DARK Lab, and was previously a software engineer, after completing an integrated masters of mathematics and computer science at Somerville College, University of Oxford.
Linqing Liu
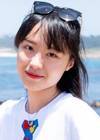
Natural Language Processing
Linqing's primary research interest lies at the intersection of Deep Learning and Natural Language Processing (NLP). She is currently working on Question Answering (QA).
Yicheng Luo
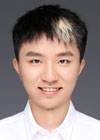
Meta-learning, Probabilistic Programming, Reinforcement Learning, Deep Generative Models
Yicheng Luo is a second Ph.D. student at UCL AI Centre supervised by Prof. Marc Deisenroth and Prof. Edward Grefenstette. He received his MEng degree from the Department of Computing, Imperial College London. For his thesis, he worked on probabilistic program analysis with Dr. Antonio Filieri.
He is interested in the design and implementation of efficient and scalable Bayesian inference methods with applications in robotics, reinforcement learning, and probabilistic programming. In particular, he is currently interested in understanding how to utilize offline reinforcement learning pretraining to achieve sample-efficient online learning.
Mirgahney Mohamed

Mirgahney H. Mohamed is a PhD student at University College London working on 3D computer vision and uncertainty estimation. He obtained his master's degree in Machine Intelligence from AMMI - African Master in Machine Intelligence at AIMS, and undergrad in Statistics and Computer Science from University of Khartoum Faculty of Mathematical Science."
Antonin Schrab
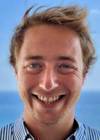
Kernel Methods, Hypothesis Testing, Generative Modelling
Antonin's research lies at the intersection of Machine Learning and Statistics. During his PhD, he has proposed methods addressing the fundamental kernel selection problem in diverse hypothesis testing settings such as the two-sample, independence and goodness-of-fit frameworks. His work is both theoretical (proving optimality guarantees for the constructed tests) and practical (providing parameter-free implementations which contribute to the wide use of these kernel tests in real-world applications). He also has a keen interest in Generative Modelling. Antonin is supervised by Benjamin Guedj and Arthur Gretton. Prior to starting his PhD, he completed the MSc Machine Learning at UCL and obtained his Master of Mathematics from the University of Oxford.Oliver Slu
mbers

Multi-Agent Reinforcement Learning
I am interested in how we can design algorithms to allow for effective agent interaction in multi-agent interactions. In particular, I work on bridging the gap between multi-agent systems and Game Theory and how we can apply the large body of Game-Theoretic techniques to interacting reinforcement learning agents. Prior to joining the CDT, I completed my BSc in Economics at the University of Warwick and also the MSc in Computational Statistics and Machine Learning at UCL.
Yuchen Zhu

Causal Machine Learning, AI robustness
Yuchen is interested in making machine learning robust through modelling causal invariances within data. She develops both theory and algorithms for unbiased causal effect inference. In her investigation, she often uses kernel methods and methods inspired by the econometrics literature. Previously, she earned her Master's degree in Machine Learning from UCL, and a Bachelor's degree in Mathematics from Cambridge. She is advised by Professor Ricardo Silva and Dr Matt Kusner.
Sicelukwanda Zwane
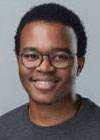
Safe Learning in Robotics
Sicelukwanda is interested in designing safe and robust algorithms for learning tasks in robotics settings. He is a member of the Statistical Machine Learning lab where he studies model-based reinforcement learning with Gaussian Processes and different approaches to incorporating safety constraints when learning robot tasks. He is supervised by Marc Deisenroth and Dimitrios Kanoulas.
19/20 academic year
Felix Biggs
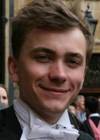
Statistical learning theory
Felix' broadly-defined research interests are focused on the mathematical aspects of learning in artificial and biological systems, particularly through the lens of statistical learning theory. His work thus far has focused on PAC-Bayes to understand learning in shallow and deep neural networks, and to obtain non-vacuous generalisation bounds and improved training methods for the above. He is advised by Benjamin Guedj and John Shawe-Taylor.
Samuel Cohen
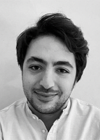
Imitation learning, time-series analysis, gaussian processes and multi-task reinforcement learning
I am a 3rd year PhD student in Prof. Marc Deisenroth’s group, and co-supervised by Prof. Julien Cornebise. Throughout my PhD I have been working on applying optimal transport tools to generative modeling, imitation learning, time-series analysis, gaussian processes and multi-task reinforcement learning. I have also been working at Facebook AI research for the past few months with Brandon Amos. Previously, I studied the MSc in Statistical Science at Oxford University and the BSc in Mathematics at Imperial College.
Augustine Mavor-Parker
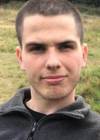
Reinforcement learning and neuroscience.
I hold an MSci degree in physics from UCL. During my undergraduate degree, I received a Royal Astronomical Society research bursary to apply machine learning to cosmology at the Mullard Space Science Laboratory with Prof. Tom Kitching. Additionally, I worked as a bioinformatics intern for Illumina, where I developed deep learning approaches for genomic analysis. In my final year I worked with Prof. Ofer Lahav, again applying deep learning to cosmology. I have also previously worked at Kagenova as a Machine Learning Research Scientist developing geometric deep learning approaches. My current research interests are geometric deep learning, sparse reward reinforcement learning and self-supervised learning. I am supervised by Lewis Griffin and Caswell Barry.
Luca Morreale
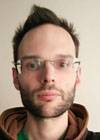
Geometry processing and Surface representation
I am a PhD student at UCL advised by Niloy J. Mitra from UCL and Noam Aigerman from Adobe Research. My research lies at the intersection of geometry processing, computer graphics and deep learning. I am particularly interested in surface representations naturally enabling geometric applications. More information on my website.
Jas Semrl
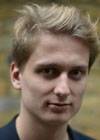
I am a PhD student in the Department of Computer Science at UCL, supervised by Professor Robin Hirsch. My main interests include Relation Algebra and its Applications in Computer Science, as well as Formal Logic more generally. I am a member of the PPLV Research Group and the Foundational AI CDT. I have received my MEng in Computer Science from UCL in 2019.
Jingwen Wang
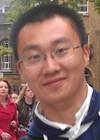
Semantic SLAM, 3D Reconstruction, Neural Scene Representations
I am a PhD student at the CDT in Foundational AI at UCL , supervised by Prof. Lourdes Agapito and Prof. Niloy Mitra. My research interest lies in object-aware semantic SLAM and 3D reconstruction, combining object-level scene understanding and SLAM systems using learning-based approaches. Prior to my PhD I obtained MRes in Robotics from UCL, and BEng in Electrical Engineering from the University of Liverpool.
Changmin Yu
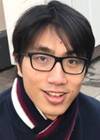
Reinforcement learning, probabilistic learning, computational neuroscience
I am a third-year PhD student supervised by Neil Burgess and Maneesh Sahani. My main research interests lie in reinforcement learning, probabilistic learning, and computational neuroscience. Prior to UCL, I studied mathematics at Imperial College and Cambridge.
Jakob Zeitler
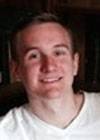
Causal Inference for Machine learning
Graduated with a BA in PPE at University of Exeter in 2017 focusing on philosophical and mathematical basis of causality. Started a Computer Science PhD at Syracuse University in New York, transferring to UCL in 2019. Currently researching scalable methods of partial identification with applications to AI fairness and healthcare.