Memristors for green AI
7 September 2020
Extremely energy-efficient artificial intelligence is now closer to reality after a study by EEE researchers found a way to improve the accuracy of a brain-inspired computing system.
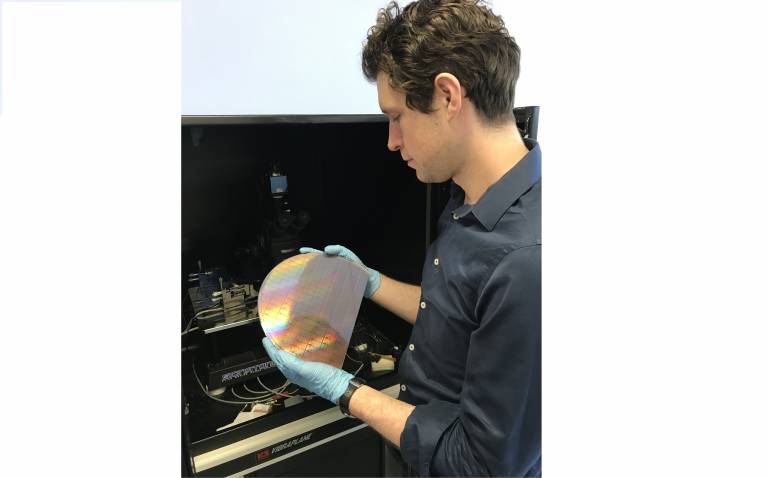
Dr Adnan Mehonic recently published a study that is looking at ways to improve the accuracy of memristor-based neural networks, the main hindrance that keeps the AI technology from being fully adopted and scaled-up.
Training one AI model in its current form would generate 284 tonnes of carbon dioxide, equivalent to the lifetime emissions of five cars. Replacing the transistors that make up all digital devices with memristors, could reduce this to a fraction of a tonne of carbon dioxide – equivalent to emissions generated in an afternoon’s drive.
Memristors electronics are 1,000 times more energy-efficient than existing AI hardware and they can pack huge amounts of computing power into hand-held devices, removing the need to be connected to the internet and allowing data processing to be local rather than sending it to remote data centres. Local processing is highly preferential if we consider privacy implications.
But until now, these systems have been prone to error due to the analog nature of information processing. Dr Mehonic found that accuracy could be greatly improved by getting memristors to work together in several sub-groups of neural networks and averaging their calculations, meaning that flaws in each of the networks could be cancelled out.
Dr Mehonic, his PhD student Dovydas Joksas (both UCL Electronic & Electrical Engineering), and colleagues from the UK and the US, tested the new approach in several different types of memristors and found that it improved the accuracy of all of them, regardless of material or particular memristor technology. It also worked for a number of different problems that may affect memristors’ accuracy due to the analog nature of information processing. Their approach increased the accuracy of the neural networks for typical AI tasks to a comparable level to software tools run on conventional digital hardware.
Dr Mehonic, director of the study, said: “We hoped that there might be more generic approaches that improve not the device-level, but the system-level behaviour, and we believe we found one. Our approach shows that, when it comes to memristors, several heads are better than one. Arranging the neural network into several smaller networks rather than one big network led to greater accuracy overall.”
Memristors are often described as a neuromorphic (brain-inspired) form of computing because, like in the brain, processing and memory takes place at the same physical location, avoiding wasteful movement of data, the fundamental inefficiency of current computer systems.
They were considered revolutionary when they were first built over a decade ago and have since been manufactured commercially in memory devices, but the research team say they could be used to develop AI systems within the next three years.
Links
- Full paper in Nature Communications
- Dr Adnan Mehonic’s academic profile
- Research Project - Next-generation adaptive electronics for neuromorphic engineering
Image
- Top: a wafer filled with memristors. Middle: Dr Adnan Mehonic.