This focus area aims to develop a strategy for 100% circularity of demolished concrete, whether as a feedstock in the cement kiln, in blended cements, or as aggregate.
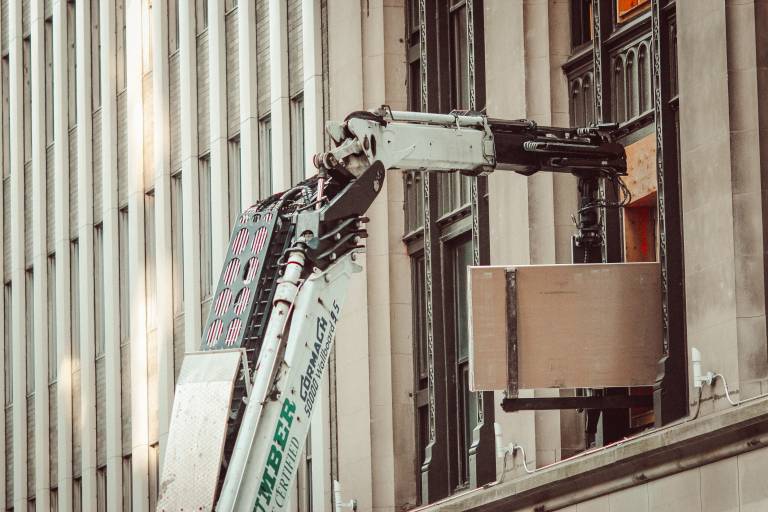
Description
The project aims to maximise the circularity potential of the construction and demolition waste (CDW), which not only includes waste concrete, but also other non-hazardous materials, such as wood, panels, metals, etc.
This project aimed at capturing the complex value generated from the circularity of CDW, which we looked not only from the economic perspective, but also from environmental, social, and technical perspective. To capture this complex value generation, we focused on the systems thinking perspective of circular economy.
Project 2E is led by Dr Soumava Boral, who is based at the University of Leeds.
This project is sub-divided into two parts:
Part I: Value optimisation of CDW by Complex System Modelling
In this part, we tried to map the whole value-chain of the CDW, from raw materials excavation to end-of-life, and thereby putting those secondary materials again into the life cycle. We tried to develop a dynamic material flow model, along with how it is impacted by several external agents (e.g., govt. policies, legislations, taxes, etc.). To do that, we adopted two modelling approaches: system dynamics and agent-based modelling and simulation. Until now, we found out the following:
- In the UK, there is a lack of proper tracking system of CDW. They are superficially reported and categorised into hazardous and non-hazardous components. Different UK Govt. statistical data just highlighted how much wastes were generated in each year, till 2018, and how much was recovered (the figure is near to 95%). But detailed description about where each type of material is used (e.g., use of recycled concrete aggregate (RCA) in fresh concrete, and/or road sub-base, etc.) are not present in the statistical reporting.
- More intensive reporting is required for dynamic modelling of the UK construction waste value-chain. This will in turn help in modelling how different circularity metrics coupled with the material flows will be impacted by these flows and will help in identifying the key leverage points in the system, to achieve the Net Zero 2050 goal.
- The generalised model is running with fictitious data, but requires more participation from industries and different government bodies to refine the model, and to perform different scenario analyses.
Part II: Capturing the complex technical value of waste concrete through explainable machine learning models
In this part, we are in the process of modelling the complex technical value of waste concrete, more specifically RCA. Until now, in literature, we found extensive studies on the application of machine learning models to predict different properties of RCA (e.g., compressive strength, splitting tensile strength, chloride resistance, creep, elastic modulus, etc.). But these literatures considered a limited set of explanatory variables to predict these properties. This is because, the data for most of the explanatory variables are not reported in previous experimental studies.
Furthermore, the response variables are complexly related with each other, and they also impact the other economic, social, and environmental circularity metrics. Additionally, the traditional machine learning models are considered as ‘black-box’ approaches, and recently machine learning communities are focusing on explainability and trustworthiness of these models.
We developed a generalised database with multiple explanatory variables and response variables. The novelties of this work are as below:
- It didn’t ignore significant explanatory variables for missing data. We employed data imputation approaches along with experts’ opinions to get the data.
- We analysed different technical properties of recycled aggregate concrete and focused rigorously on model explainability and trustworthiness.
- We tried to find out ‘why’ each of the machine learning models perform better in terms of performance compared to others.
We discovered the following:
- When RCA is incorporated in the RAC, although the embodied carbon decreases along with the increase of RCA percentage, the carbon intensity index (viz., to maintain the same strength of RAC, we need to put more amount of cement. And per tonne of cement production generates around 800 Kgs. of CO2) increases.
- The total cost of the RAC production decreases, along with the increase of the RCA percentage. But the rate of decrease of cost intensity index is much lower than the total cost. This is because, to compensate the strength reduction, more cement is used in the mixes, which in turns increases the price.
We are currently in the process of performing the following:
- Joint prediction of multiple output parameters and quantifying their causal dependencies through explainable machine learning models.
- Joint optimisation of technical, environmental, economic and societal circularity metrics.