Associate Professor of Chemical Engineering
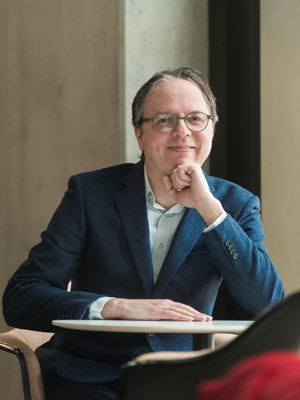
Biography
Federico Galvanin received the degree in Chemical Engineering in 2006 and the Ph.D. degree in 2010 at the University of Padova. After a post-doctoral activity in Padova focusing on the optimal design of clinical tests for the identification of physiological models, he has been working as a post-doc Research Associate at University College of London (UK) since 2014.
In September 2015 he started a new position as a Lecturer at the UCL Department of Chemical Engineering, where he became Associate Professor in 2021. His main areas of expertise are in the field of model identification including optimal design of experiments (theory and applications) for the development of chemical, biological and physiological models. In particular, his research activity is related to i) the development of advanced techniques for the optimal model-based design of experiments (MBDoE) in dynamic systems; ii) the application of conventional and advanced design of experiments techniques to physiological systems as well as to chemical processes; iii) the development and online application of model identification techniques in automated systems.
He is leading the Galvanin System Identification Group at UCL. In the group, a systems-based approach is employed for the design of optimally informative experiments with the goal of identifying dynamic models with the minimum cost, time and effort in the experimentation.
Teaching Summary
Dr Galvanin's departmental teaching activities are mainly in the areas of process design and simulation of chemical processes. Below are the modules he is currently involved in teaching.
- CENG0025 Process Systems Modelling and Design
- CENG0017 Process Dynamics and Control
Research Summary
Galvanin's research interests lie at the interface between mathematical modelling and experimentation. The research activity is particularly focused on the use of advanced computational tools for the development of predictive models for the characterisation of complex systems in both chemistry and physiology.
Dr. Galvanin has a specific expertise in the following areas:
- Optimal experimental design for model identification
- Kinetic modelling, chemical reactor modelling
- Pharmacokinetics/pharmacodynamics modeling
- Modeling of physiological systems
- Machine learning-assisted model identification
- Digital chemical manufacturing
The current research activity is primarily focused on the optimal design of experiments for i) the identification of kinetic models in microreactor platforms; ii) the development of pharmacokinetic models for the detailed study of physiological systems.
Awards
2014
Awarded the “Process Systems Enterprise Ltd. Model-based Innovation Prize 2014" for the paper: F. Galvanin, M. Barolo, R. Padrini, S. Casonato, F. Bezzo (2014). A model-based approach to the automatic diagnosis of Von Willebrand disease. AIChE J., 60, 1718-1727.
2012
Awarded as runner-up in “Process Systems Enterprise Ltd. Model-Based Innovation Prize 2012" for the paper: Galvanin, F., M. Barolo, F. Bezzo (2012). On the use of continuous glucose monitoring systems to design optimal clinical tests for the identification of type 1 diabetes models. Computer Methods & Programs in Biomedicine., 109, 157-170.
2009
Awarded as runner-up in “Process Systems Enterprise Ltd. Model-Based Innovation Prize 2009" for the paper: Galvanin, F., M. Barolo and F. Bezzo (2009). Online model-based redesign of experiments for parameter estimation in dynamic systems. Ind. Eng. Chem. Res., 48, 4415-4427.
Education
2010
Chemical Engineering, University of Padova, Italy
2006
Chemical Engineering, Master’s degree, University of Padova, Italy
Professional Affiliations
- MIChemE – member of IChemE
- MAIChE – member of AIChE
- FHEA – member of HEA
Publications
Huang, C., Cattani, F., Piccione, P. M., & Galvanin, F. (2022). A stochastic modelling approach for the characterisation of collision exchange processes. Chemical Engineering Science, 117207. doi:10.1016/j.ces.2021.117207
Quaglio, M., Roberts, L., Bin Jaapar, M. S., Fraga, E. S., Dua, V., & Galvanin, F. (2020). An artificial neural network approach to recognise kinetic models from experimental data. Computers & Chemical Engineering, 106759. doi:10.1016/j.compchemeng.2020.106759
Quaglio, M., Waldron, C., Pankajakshan, A., Cao, E., Gavriilidis, A., Fraga, E. S., & Galvanin, F. (2019). An online reparametrisation approach for robust parameter estimation in automated model identification platforms. Computers and Chemical Engineering. doi:10.1016/j.compchemeng.2019.01.010
Galvanin, F., Barolo, M., Padrini, R., Casonato, A., & Bezzo, F. (2014). A model-based approach to the automatic diagnosis of von Willebrand disease. AIChE Journal, 60 (5), 1718-1727. doi:10.1002/aic.14373
Galvanin, F., Macchietto, S., & Bezzo, F. (2007). Model-Based Design of Parallel Experiments. Industrial & Engineering Chemistry Research, 46 (3), 871-882. doi:10.1021/ie0611406