UCL Computer Science wins top prize in Kuopio Tomography Challenge 2023
12 January 2024
Working with the University of Bremen, the UCL Computer Science team clinched victory in the Kuopio Tomography Challenge. Their winning approach combined deep learning techniques with classical methods for the nonlinear inverse problem in Electrical Impedance Tomography (EIT).
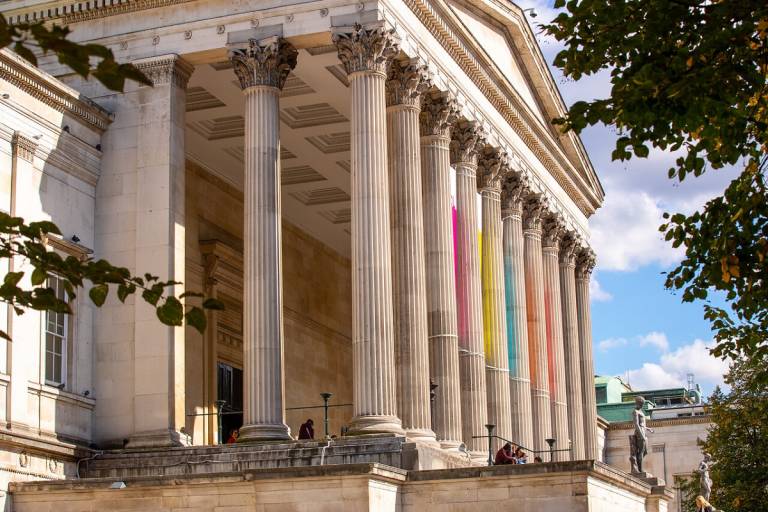
The UCL Computer Science team, in collaboration with esteemed researchers from the University of Bremen, claimed first place in the Kuopio Tomography Challenge 2023 (KTC2023), organised by the Finnish Inverse Problems Society (FIPS).
The competition, open to global scientists and research groups, centred around electrical impedance tomography (EIT) – a technique that is used to estimate the distribution of conductivity within an object. The EIT inverse problem reconstructs the conductivity distribution from measurements of voltage on the boundary of the object. These measurements are stimulated by applying a small, harmless, electrical current on the boundary of the object. Medically, EIT has been proposed for the non-invasive detection and monitoring of disease.
The teams competing in KTC2023 were tasked with developing algorithms capable of accurately reconstructing the shapes of 2D targets imaged using EIT.
The UCL-Bremen team not only excelled in overcoming the challenge of working with incomplete boundary measurements but also segmented their reconstructions into three components: water, resistive inclusions, and conductive inclusions.
Their success was celebrated with an invitation to a minisymposium at the Inverse Days Conference in Finland as well as the Grand Prize – the Ultimate Electric Measurement Device, a vintage multimeter.
Commenting on the win, Professor Simon Arridge said: "The Kuopio Tomography Challenge is an annual challenge now in its 3rd year, which seeks to find practical solutions to real-world inverse problems of varying difficulty.
This year we joined with colleagues in Bremen to tackle the difficult non-linear problem of EIT, using a combination of deep learning and classical approaches, sometimes referred to as 'Physics-informed deep learning'. Winning the challenge represents a significant advance for such hybrid techniques, which are likely to become increasingly important as AI is introduced into real real-world problems where training data is limited."