MSc Machine Learning Paper Receives International Acclaim
8 October 2019
MSc Machine Learning students achieved double success with published research paper; “SentiMATE: Learning to play Chess through Natural Language Processing”, presented at AIIDE-19. It also received a write-up in MIT Technology Review.
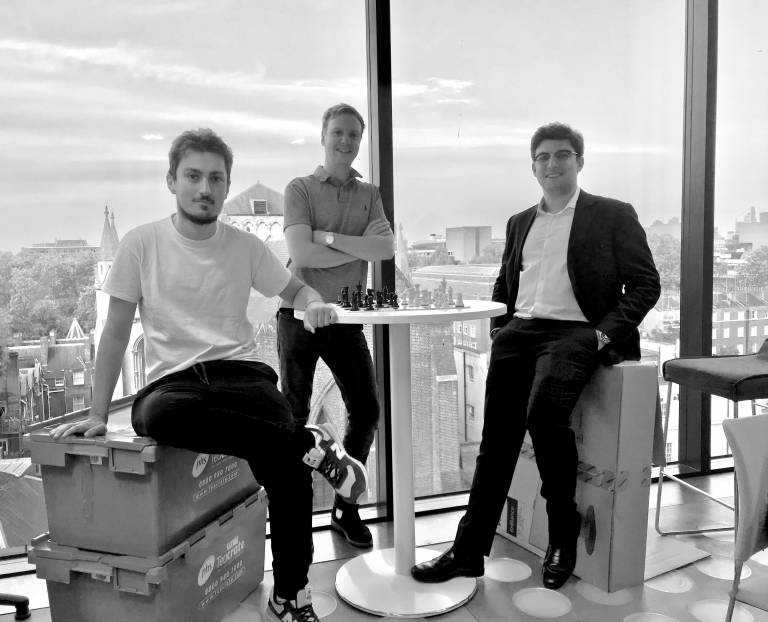
MSc Machine Learning students achieved double success when they published their research paper; “SentiMATE: Learning to play Chess through Natural Language Processing”. Not only was the paper accepted for an Oral Presentation at the AIIDE-19 Workshop on Artificial Intelligence for Strategy Games, it also received a write-up in MIT Technology Review.
The team developed a novel deep learning model named SentiMATE that was able to learn to play chess from text-based commentary, as well as some key strategies in the game. The idea initially came about as the team felt that learning strategy from text-based data could be both an interesting and important research avenue to explore. The team gathered large quantities of chess move commentaries from various books, blogs and websites into a unified raw dataset. Using state of the art models within NLP and Deep Learning they managed to learn an evaluation function which could effectively measure the quality of a given move. This was in turn was used to build an innovative chess engine.
Students Isaac Kamlish, Isaac Bentata Chocron and Nicholas McCarthy were delighted to receive the news: “We are overwhelmed by the positive reception from the international AI and Chess Community”.
The work was developed as part of Statistical NLP course run by Professors Sebastian Riedel and Tim Rocktäschel -
“I am sure many of us have heard about the accomplishments of reinforcement learning agents that learn from reward when acting in an environment. While very successful for games such as Go, Chess and StarCraft II, this approach unfortunately requires vast computational resources. In our NLP course, a group of MSc students developed a proof-of-concept method for utilizing the sentiment of Chess commentaries to train agents in a more efficient way. Finding ways to learn from such textual human domain knowledge holds the key for unlocking novel, more real-world applications for reinforcement learning, and I am impressed that these students contributed to this emerging field by publishing in the AIIDE-19 Workshop. I also believe this accomplishment demonstrates the excellence of our UCL MSc program and its students."
Sebastian Riedel went on to say:
"A central pillar of our module is the idea of teaching students to build actual NLP systems, not just only about them in theory. To this end we also assess them from this perspective. There are no exams. Instead, they have to define and scope a problem---one of the hardest parts—solve it and present it. The SentiMATE team has done a fantastic job at this, but they are not alone. Every year the UCL MSc students impress us with their skills, engagement and creativity."
The students see the work opening up various opportunities within AI research: “We hope that this work inspires more research that leverages text-based information to learn successful agent policies” said the SentiMATE team. “There is an abundance of books, blogs and papers all waiting to be learnt from”.